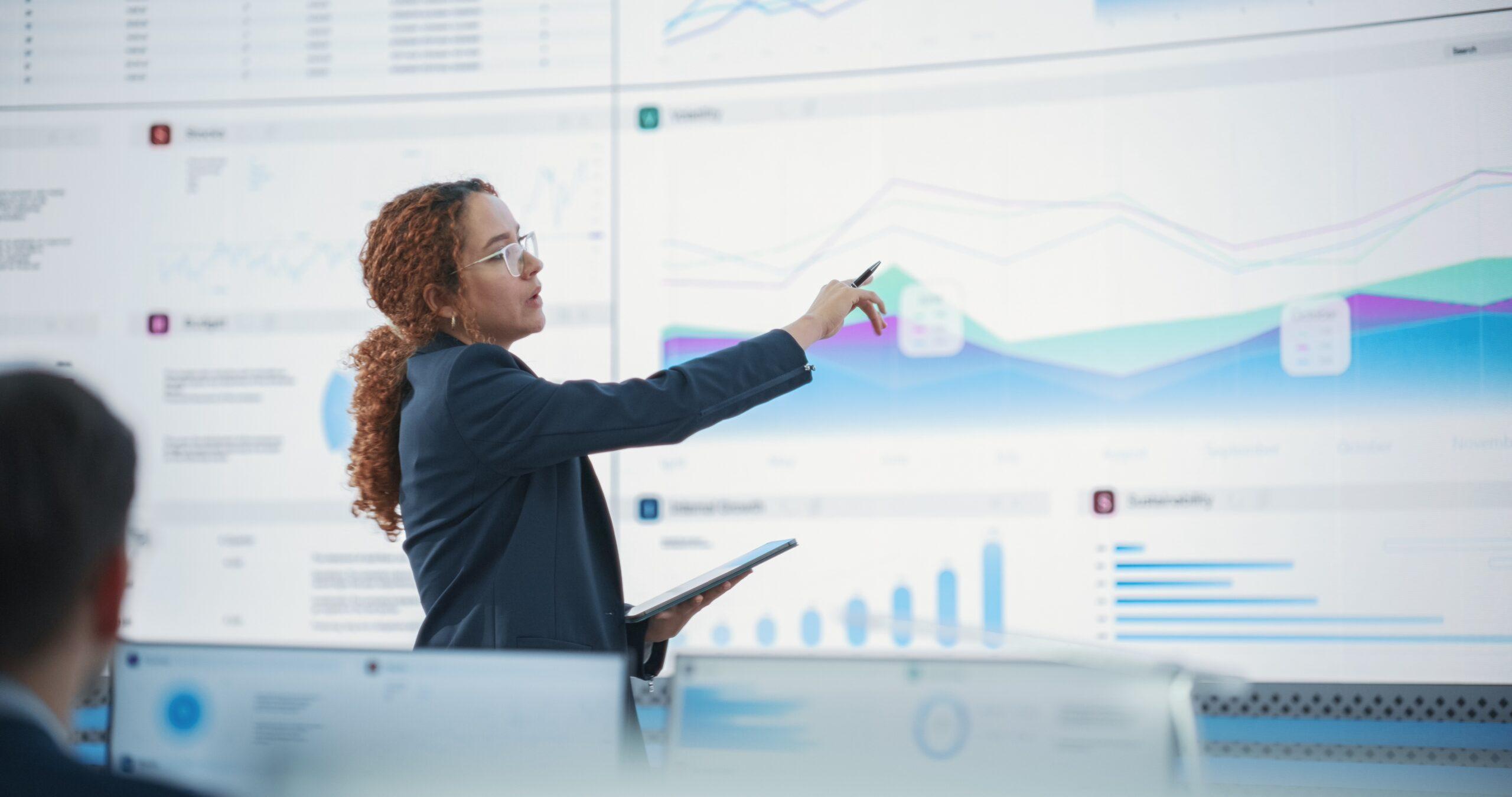
Hiring + recruiting | Blog Post
Data Scientist vs. Data Analyst: Unlocking the Power of Information
Laura Berlinsky-Schine
Share this post
Data-driven occupations are exploding. As businesses increasingly recognize the importance of deriving insights from the abundance of data at their disposal, the demand for professionals in this sphere is growing exponentially. The World Economic Forum’s Future of Jobs Report 2025 finds that technology-related roles, including big data specialists, are the fastest-growing jobs. The report also reveals that big data itself is one of the fastest-growing skills, along with AI.
Here, we will explore two of the most well-known data roles—data scientist vs. data analyst—and discuss how each can benefit your business and give you a competitive advantage.
A Data-Driven Culture
“Data readiness is the foundation that ensures that good-quality data is readily available to the right person at the right time,” writes Ganes Kesari in MIT Sloan Management Review.
Today, there is an enormous emphasis on building a data-driven culture. That’s an environment where data is deeply ingrained into the principles of the organization, driving all decisions and processes.
The benefits of creating a data-driven culture are numerous: it drives automation and consistency, helps companies gain a better understanding of how to proceed and what the results will be, increases profits, improves efficiency and productivity, and fosters strategic decision-making. Businesses across industries with a data-driven culture gain a competitive edge.
Consider the healthcare sector. Data professionals can identify patterns in patient and organizational data to predict health conditions and disease outbreaks. This allows them to better allocate funds and alter operations accordingly.
While a data-driven culture requires transparency, buy-in, and understanding from everyone in the organization, it starts with hiring the right data professionals. Data scientists and data analysts are central to building this type of business environment.
Data Analyst vs. Data Scientist: Responsibilities
Data Analyst
Using a variety of technologies and tools, data analysts solve problems with data. They obtain data from various sources, query databases, and clean data for analysis, addressing inaccuracies and inconsistent data.
Data analysts then analyze and interpret data to identify trends, patterns, and anything else noteworthy. This is called data analytics. They translate these findings into a digestible format, such as dashboards, reports, or data visualizations, for various stakeholders.
These professionals focus on deriving meaning from past or existing data, working with stakeholders to understand business challenges and find solutions through data. This allows them to draw meaningful conclusions from the information.
Data Scientist
Like data analysts, data scientists obtain and clean raw data. However, they focus on the future, predicting trends using advanced techniques.
This is a multifaceted and complex process. Data scientists may devise machine learning algorithms or predictive models, architect and create tools and methods for analyzing data, and develop data visualization tools, reports, dashboards, and more.
As with data analysts, data scientists aim to solve business challenges. They evaluate data, searching for hidden insights and discovering patterns. But unlike their counterparts, they make predictions about their findings. Their focus is on creating new ways to uncover data and derive meaning from it.
Skills Required
Data analysts and data scientists require some overlapping skill sets. For example, they need to have a strong background in statistics and complex problem-solving. They should also have soft skills, including communication so they can articulate their findings to stakeholders and the ability to collaborate with team members. Creativity, curiosity, critical thinking, and analytical skills are also essential.
However, some skills are particular to the role. Here’s a breakdown of the skills necessary for a data scientist vs. data analyst.
Data Analyst
Data analysts must have experience with programming languages like R, SQL, and Python. They should also have knowledge of data visualization and business intelligence software, such as Tableau and Power BI, as well as a comprehensive understanding of the concepts involved. Data management—collecting and organizing data—is an essential skill at the core of a data analyst’s role. They must also be able to work with databases and make predictions.
While machine learning is not a central skill for data analysts, having a basic understanding of the technology can help inform their work and give them a competitive edge.
Data Scientist
Data scientists typically need to have all of the skills of a data analyst and then some. For example, they must have comprehensive knowledge of object-oriented programming (OOP) languages. They should also be well-versed in big data platforms and technologies like Hadoop, MySQL, Spark, and TensorFlow.
Data scientists must have thorough experience with predictive analytics and data modeling. An increasingly critical skill in the data science space is machine learning. Unlike data analysts, data scientists should have more than a cursory understanding of ML. Their experience with AI/ML allows them to create models that can learn and adapt independently without manual manipulation. Deep learning, a subset of machine learning, also helps them create more sophisticated models.
Data Scientist vs. Data Analyst: Career Potential
While both roles deal with turning data into actionable insights, data analysts are focused on the past and present, while data scientists make predictions about the future.
The role of data scientist is a more advanced and technical job and usually more lucrative. According to Glassdoor, the median total pay for data analysts is $112,000, while the median total pay for data scientists is $167,000 in the US. Because the latter occupation has higher earning potential and requires more advanced skills, many data analysts end up pursuing data scientist roles.
Ultimately, both roles help foster a data-driven culture. They are critical for improving the organization and utilizing data to drive efficiency and increase profits.
Looking to hire a data analyst or data scientist? Whether you need freelancers, full-timers, or an entire team, we can help. Hire pre-vetted professionals in Latin America, Canada, or Europe from Terminal’s elite talent pool. Get started by browsing elite developers for free.